I am a postdoctoral researcher at the University of Maryland (UMD), College Park, with Dr. Abhinav Shrivastava.
My core interest lies in video analytics, specifically designing scalable solutions from the current state-of-the-art frameworks. Motivated by my research and memory networks, I wish to design a framework to mimic human intelligence for sequential modeling. I am also interested to explore about human behavior and neuroscience to demystify human intelligence.
I am a Ph.D. candidate in the Department of Computer Science, IIIT Delhi, India, advised by Dr. Chetan Arora (IIT Delhi). My research, supported by the Visvesvaraya Ph.D. Scheme for Electronics & IT (Govt. of India), focuses on analyzing day-long egocentric videos using deep learning and statistical frameworks. My thesis is centered around designing scalable solutions for days to weeks-long egocentric lifelogs from state-of-the-art sequential modeling frameworks in a purely unsupervised setting. I have demonstrated that the state-of-the-art deep learning frameworks viz RNN, LSTM, IndRNN, and the recently introduced Transformer network failed to model massive long sequences. My research is significant feat to address the scalability issue in recent works and establish the state-of-the-art. We are the first to work on the Disney (comprises 4 to 8 hrs long video samples) and UTE datasets (comprises 3 to 5 hrs long video samples) for summarization and temporal segmentation. All the solutions are scalable to thousands of time steps and completely unsupervised/self-supervised. Currently, we are working on the EgoRoutine photostream dataset (which scales up to 20 days).
You can contact me via email at pnagar (at) umd (dot) edu.
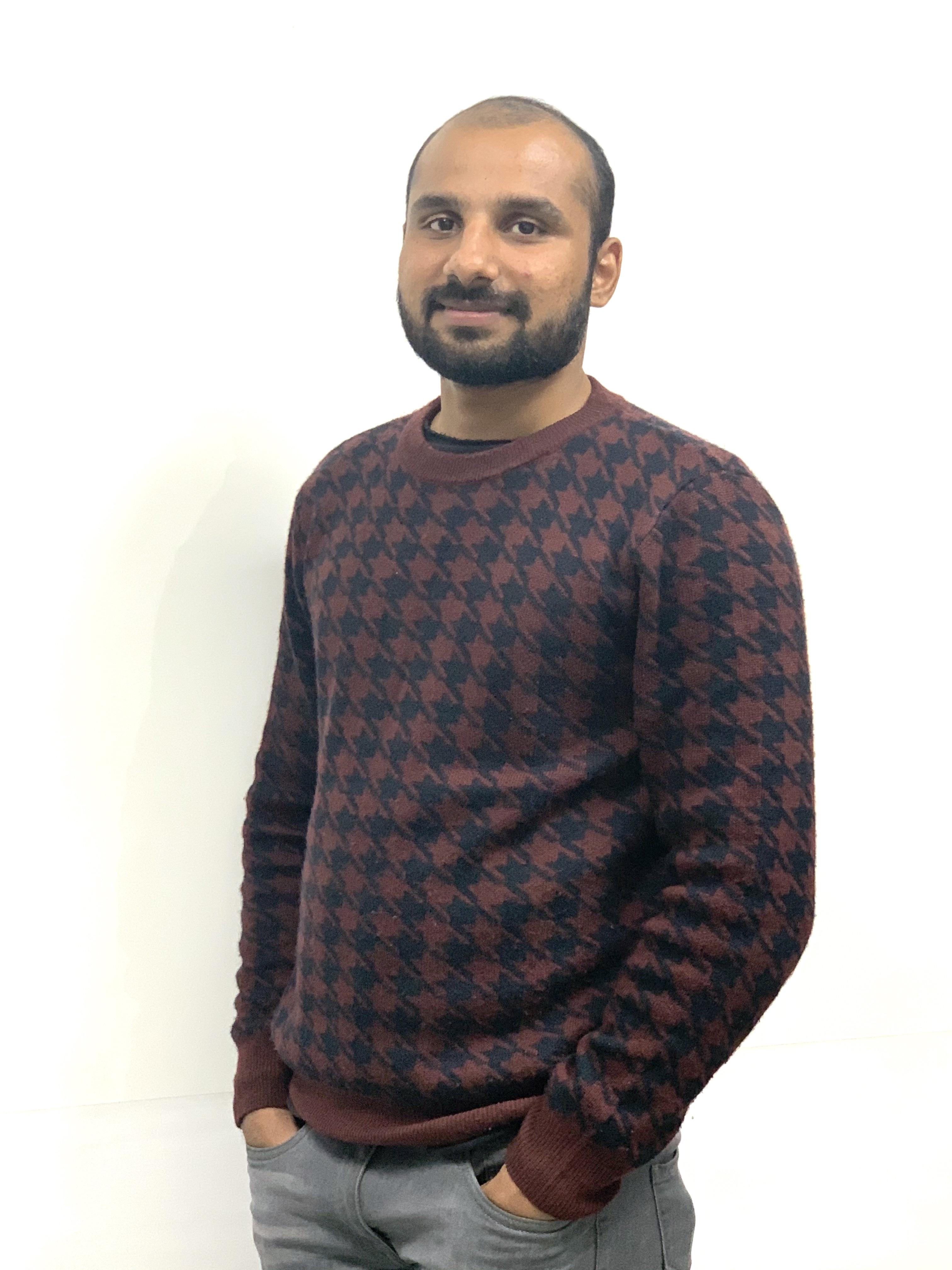