SEMA: Semantic Attention for Capturing Long-Range Dependencies in Egocentric Lifelogs
Abstract
Transformer architecture is a de-facto standard for modeling global dependency in long sequences. However, quadratic space and time complexity for self-attention prohibits transformers from scaling to extremely long sequences (> 10k). Low-rank decomposition as a non-negative matrix factorization (NMF) of self-attention demonstrates remarkable performance in linear space and time complexity with strong theoretical guarantees. However, our analysis reveals that NMF-based works struggle to capture the rich spatio-temporal visual cues scattered across the long sequences resulting from egocentric lifelogs.
To capture such cues, we propose a novel attention mechanism named SEMantic Attention (SEMA), which factorizes the self-attention matrix into a semantically meaningful subspace. We demonstrate SEMA in a representation learning setting, aiming to recover activity patterns in extremely long (weeks-long) egocentric lifelogs using a novel self-supervised training pipeline. Compared to the current state-of-the-art, we report significant improvement in terms of (NMI, AMI, and F-Score) for EgoRoutine, UTE, and Epic Kitchens datasets. Furthermore, to underscore the efficacy of SEMA, we extend its application to conventional video tasks such as online action detection, video recognition, and action localization.
Architecture
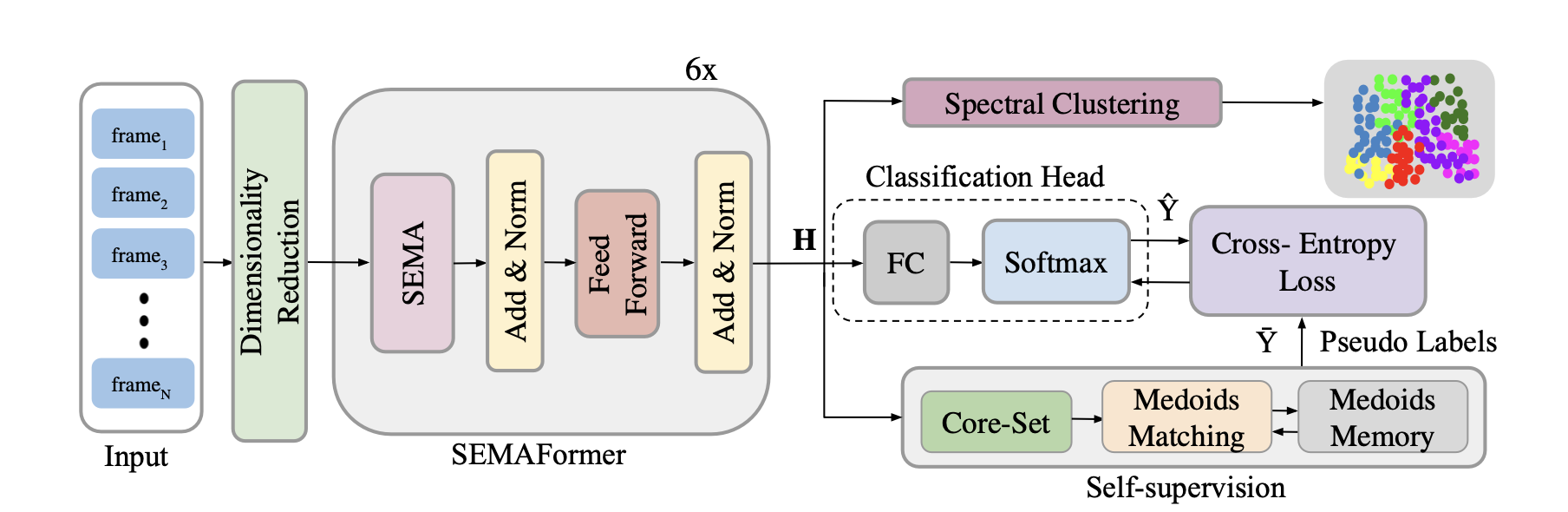
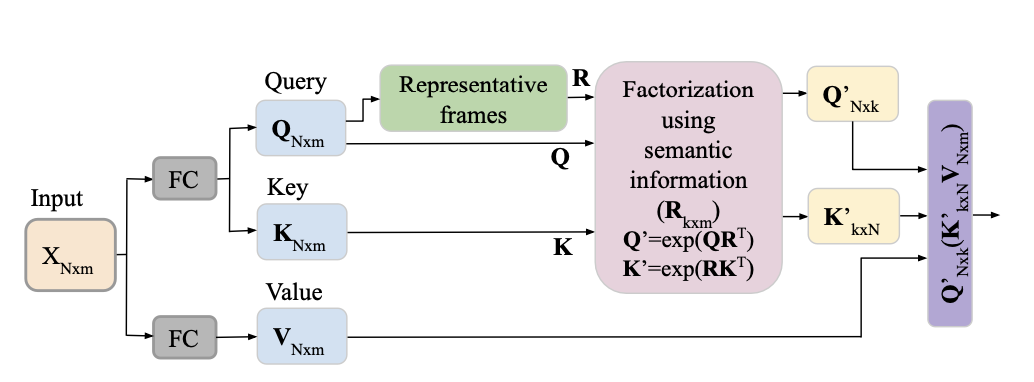
Datasets
This work uses several benchmark datasets including EgoRoutine, UTE, and Epic Kitchens for evaluating SEMA’s performance.
Code
Find the code implementation on GitHub. The implementation is based on the PyTorch library.
Supplementary Material
The supplementary material can be found here